Datasets
Neuromorphic and standard datasets for computer vision, computation, and learning tasks
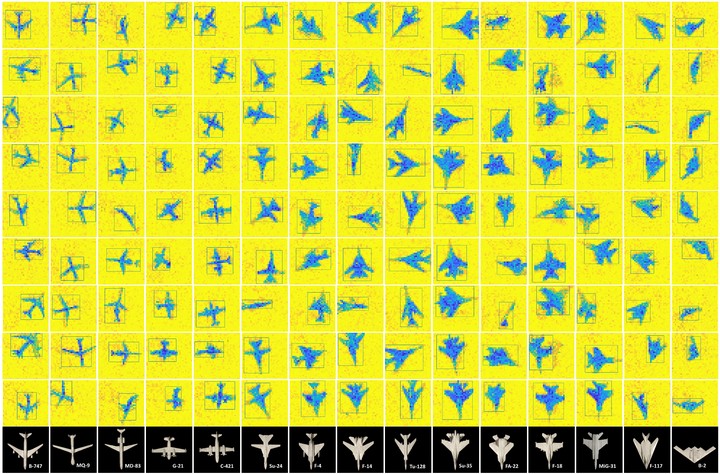
Table of Contents
Introduction
Datasets are an integral part of science. They are the means by which we can assess and, more importantly, compare and contrast the performance of our research. Although neuromorphic datasets have their limits, they are critical to neuromorphic systems and we have produced a number of datasets over the years to validate, test, and characterize the algorithms and hardware that we build. These datasets were often created to address a certain need or to test a specific aspect of a neuromorphic system.
In the field of neuromorphic engineering, datasets also play another pivotal role. They allow people to access data without needing the specialized hardware used to create and generate it. Although event-based neuromorphic sensors are becoming more widely available, they are still expensive and difficult to use. A dataset, by contrast, can just be downloaded and accessed, allowing those more interested in the algorithms and processing of the data to avoid having to fiddle with electronics and prototype hardware.
We are actively working to make this data more widely available and easier to use. We welcome any suggestions and comments.
Datasets
The EMINST Dataset
An extension of the MNIST dataset in the same format and containing letters and thousands more digits.
The MNIST dataset has become a standard benchmark for learning, classification and computer vision systems. Contributing to its widespread adoption are the understandable and intuitive nature of the task, its relatively small size and storage requirements and the accessibility and ease-of-use of the database itself.
The MNIST database was derived from a larger dataset known as the NIST Special Database 19 which contains digits, uppercase and lowercase handwritten letters. This paper introduces a variant of the full NIST dataset, which we have called Extended MNIST (EMNIST), which follows the same conversion paradigm used to create the MNIST dataset.
The result is a set of datasets that constitute a more challenging classification tasks involving letters and digits, and that shares the same image structure and parameters as the original MNIST task, allowing for direct compatibility with all existing classifiers and systems. Benchmark results are presented along with a validation of the conversion process through the comparison of the classification results on converted NIST digits and the MNIST digits
A more detailed explanation and discussion of the EMNIST dataset can be found here.
The N-MNIST Dataset
An event-based version of the popular MNIST dataset, created with saccade-like motions.

The Neuromorphic MNIST (N-MNIST) is an event-based variant of the MNIST dataset, containing event-stream recordings of the full 70,000 digits (60,000 training digits, and 10,000 testing digits). The digits were converted to events using a physical event-based camera that was moved through three saccade-like motions. The field-of-view of the camera was cropped to 34 x 34 pixels around the centre of digit and the recordings contain precise times at which each saccade begins. The N-MNIST digits are larger than the original 28 x 28 pixel MNIST digits to account for the induced motion created by the saccades. The biases of the event-based camera were pre-configured to set value, and the lighting conditions were controlled to ensure consistency across each recording.
A similar dataset, known as MNIST-DVS, also contains event-based representations of the MNIST digits but differs slightly in the way that the data was collected. Both datasets use a physical event-based camera pointed at an LCD monitor in order to display and record each digit. The difference is that MNIST-DVS moves the digit on the screen, whilst N-MNIST keeps the digit stationary and rather moves the camera to generate the motion (and therefore the events). Both methods are affected by the refresh rate of the LCD screen, although this may be more noticeable in the MNIST-DVS dataset as the image on the screen is actively changing whist the event-based camera is recording. These effects have been well characterized and efforts taken to mitigate the effect on the data recordings, however providing an alternative approach was a driving factor in the creation of the N-MNIST dataset.
The original N-MNIST dataset can be found here, and a version repackaged as an HDF5 file can be found here.
The DVS Planes Dataset
A real-world event-based vision dataset for high-speed detection and classification algorithms.
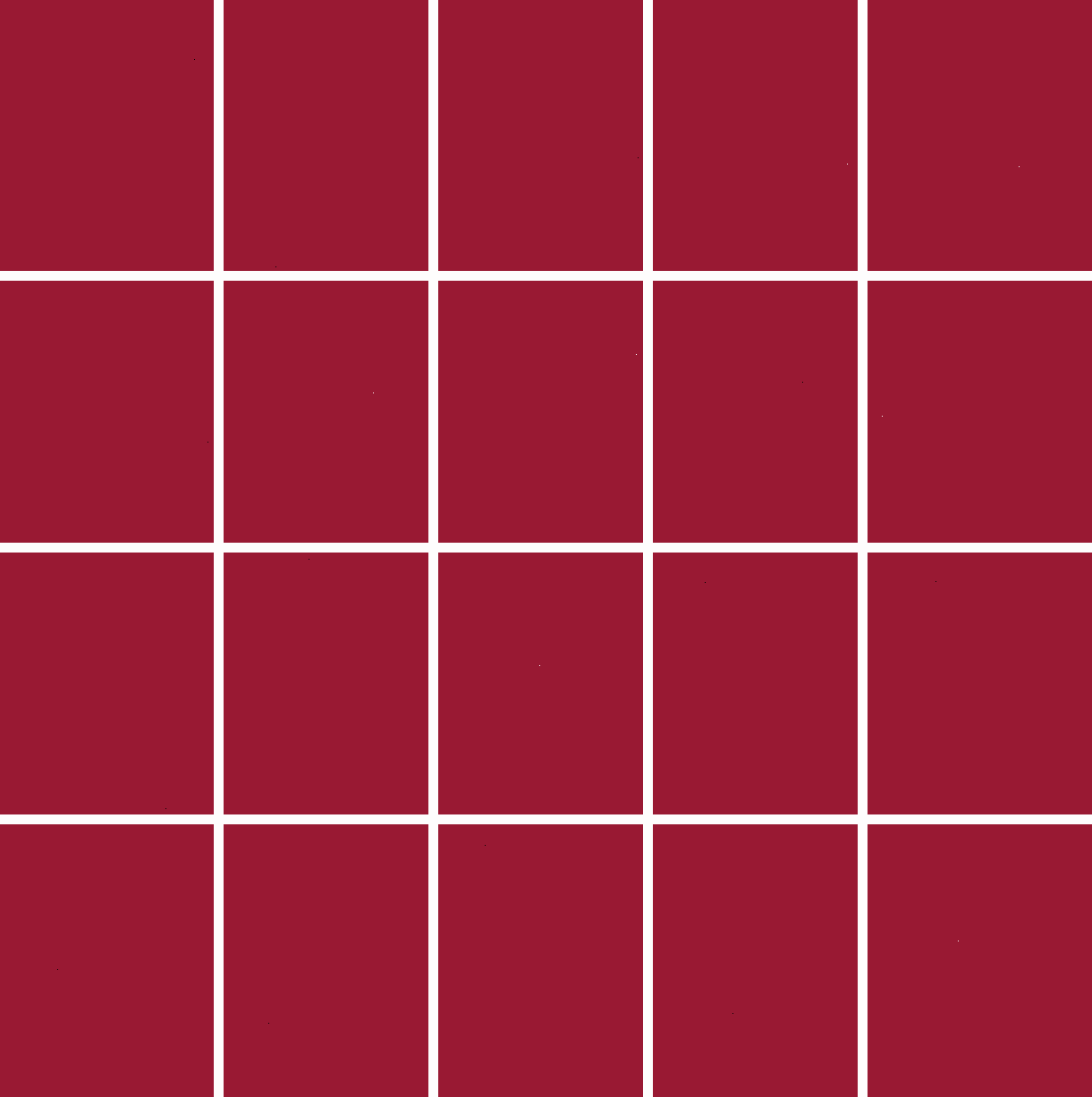
The DVS Planes dataset (formerly called the ATIS Planes dataset) is an event-based dataset of free hand dropped airplane models. The recordings were captured using an early event-based camera (known as the ATIS camera 1) and the same acquisition software used in capturing the N-MNIST dataset. The airplanes were dropped free-hand, and from varying heights and distances from the camera. Four model airplanes were used, each made from steel and all painted uniform grey. The airplanes are models of a Mig-31, an F-117, a Su-24, and a Su-35, with wingspans of 9.1 cm, 7.5 cm, 10.3 cm, and 9.0 cm, respectively.
The field of view is 304 x 240. There are 200 files per plane type making up 800 files in total. The models were dropped from a distance ranging from 120 cm to 160 cm above the ground and at a horizontal distance of 40 cm to 80 cm from the camera. This ensured that the airplanes passed rapidly through the field of view of the camera, with the planes crossing the field of view in an average of 242 ms ± 21 ms. No mechanisms were used to enforce consistency of the airplane drops, resulting in a wide range of velocities from 0 to greater than 1500 pixels per second.
This dataset was initially created to allow us to test and showcase a high-speed end-to-end classification task on a real-world dataset. You can find our paper, Investigation of Event-Based Surfaces for High-Speed Detection, Unsupervised Feature Extraction, and Object Recognition, which covers the methodology used to create this dataset in detail.
ICNS Star Tracking Datasets
The ICNS Star tracking data is now available and details can be found here. You can also access the files directly.
Example Data
Eiffel Tower Example Data

This is a short recording of a small model of the Eiffel tower from an event-based camera moved free-hand around the object. This recording was captured using an early prototype event-based sensor with a resolution of 304 x 240 pixels, and is distributed as a Matlab Matfile which can easily be loaded in Matlab or Python (via the SciPy loadmat function).
The Matlab version of this recording can be downloaded here.
- Posch, C., Matolin, D., & Wohlgenannt, R. (2011). A QVGA 143 dB Dynamic Range Frame-Free PWM Image Sensor With Lossless Pixel-Level Video Compression and Time-Domain CDS. IEEE Journal of Solid-State Circuits, 46(1), 259–275. https://doi.org/10.1109/JSSC.2010.2085952 ^